The era of the Smart Factory and Industry 4.0 may be here, but production downtime still casts a shadow over most manufacturing facilities, oil refineries and water treatment plants. Indeed, almost every factory loses 5% of its productivity capacity through faulty equipment, a loss of value that for the automotive sector alone is worth 28 million euros daily.
The mission to tackle the challenge of downtime is ongoing. However, it has progressed significantly in recent years, driven by Big Data. We are now flooded with data from a variety of sources that tell us far more about the inner workings of assets and equipment. The challenge remains making sense of, and integrating, all the intelligence now available to enhance financial and operational performance, says Alessandro Chimera, industry consultant at TIBCO Software.
The reactive approach
Looking back at the evolution of maintenance, it’s clear that we have come a long way – from a starting point of no data insight at all. For example, for the average factory of the 1980s, mechanical wear and tear routinely brought operations to a standstill. As the production line lay idle until the fault was diagnosed and repaired, costly and frustrating downtime was incurred.
With no immediate insight into the causes of the problem, and an entirely reactive approach to maintenance, the average uptime and asset reliability was just 50%, a situation that reduced productivity and had the capacity to harm brand and reputation.
Taking control: Scheduled maintenance
At the turn of the 21st century, manufacturers could no longer afford the disruption caused by random breakdowns. Greater competition and regulation demanded more reliable asset management and signalled the introduction of planned or scheduled maintenance. This meant solving a problem before it had the chance to occur or escalate, with regular scheduled services aimed at extending the lifespan of systems or equipment.
Some may say it was over zealous; regular premptive checks meant production would still grind to a halt and, while expected, this remained costly and disruptive. As such, attention turned to the need to better understand the context behind machine faults to drive a more targeted and measured response to maintenance.
Data-driven proactive maintenance
As a tool to identify the root causes of failure and apply the learning to avoid a repeat, data propelled in a new era of proactive maintenance. Machine sensors measuring factors such as temperature, pressure, flow, voltage or current, began to unlock the inner workings of equipment. This drove the emergence of Root Cause Analysis, which applied a problem-solving methodology to determine which factors represented the most effective means to predict machine failure. Meanwhile, statistical process monitoring and defect elimination strategies also came into play.
Predictive maintenance
In today’s fast and complex operating environment, where even smallest delay can see a loss of competitive advantage, we need to go a stage further. It’s why predictive maintenance has emerged as the game changer, enabling industrial companies to anticipate maintenance requirements and respond accordingly to avoid both breakdowns and maintenance delays.
Here, machine learning and streaming analytics combine, as connected real-time data brings visibility to the inner workings of processes, providing a level of insight that drives more intuitive and timely management. Equipment maintenance can now be optimised in real-time and with minimal disruption, as risks and production faults are addressed before they escalate.
Contextual operational and environmental data – captured on-site from IoT sensors – informs behavioural models, which can predict breakdowns and machine reliability, based on factors such as operating conditions and optimum detection points. Furthermore, analytic functionalities that drill into the data and visualise what is hidden, are a superior way of understanding and interpreting the information for a full picture.
As a result, the overall equipment effectiveness and uptime rises to a far more respectable 90%. Further value is added through the integration of other capabilities, such as geolocation, to identify the nearest technical support person available to attend to the issue.
Self-optimisation: The way forward
Looking to the future, we’ll see a factory floor in which IoT-enabled machines and systems can self-diagnose and make automated adjustments in response to both internal and external events, continually adapting to their environment.
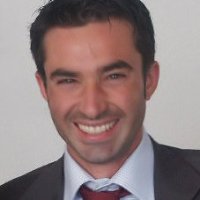
Aside from reducing the need for manual intervention, it is clear how such capabilities also offer the agility needed to respond to the unplanned ebbs and flows of the factory, e.g. the machine that ‘knows’ to expand production as sales volume increases.
As such, both predictive maintenance and self-optimisation will represent the two largest growth areas in the Internet of Things through to 2020. Traction is set to build more substantially across healthcare, transport and logistics, as more sectors embrace the opportunity that comes from augmented operational real-time intelligence. Perhaps a downtime-free future is within our reach.
The author of this blog is Alessandro Chimera, industry consultant at TIBCO Software
Comment on this article below or via Twitter: @IoTNow_OR @jcIoTnow