The fusion of Artificial Intelligence (AI) and the Internet of Things (IoT) has ushered in a new era of ultra-low-latency data processing and decision-making.
These two transformative technologies converging into a new paradigm, the Artificial Intelligence of Things (AIoT). The AIoT industry is projected to reach a total value of $24.9bn by 2028 at a CAGR of 37.7%
Edge AI combined with IoT involves running AI algorithms locally on a hardware device instead of transmitting data to a centralised server or cloud for processing.
The result? Low-latency IoT solutions that deliver speed, security and stability, even in the most demanding of scenarios.
This article delves into the integration of edge AI in IoT.
Edge AI and AIoT: a technological symbiosis
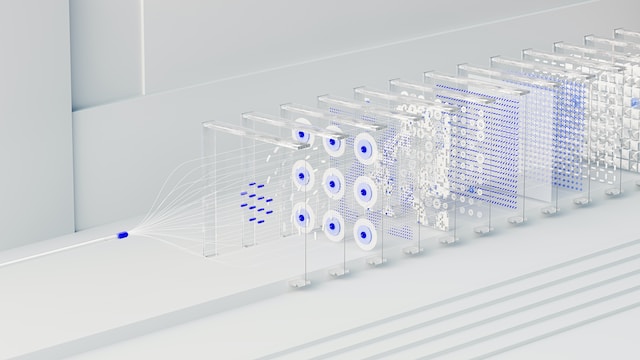
Edge AI combined with IoT leverages machine learning (ML) algorithms to locally process the data produced by IoT sensors.
This means data is processed near where it’s produced instead of being transmitted over the internet to a data centre or cloud for processing.
To delineate any confusion surrounding these acronyms, AIoT is a more general combination of AI and IoT technologies, whereas edge AI refers to deploying ML models to the edge. Edge AI combined with IoT is a form of AIoT.
Transmitting IoT data to external logic and decision-making systems is time-consuming and prevents real-time data processing – edge AI solves that issue. Edge AI deploys decision-making algorithms at the edge for ultra-low-latency IoT applications.
Examples of edge AI applications
Integrating AI systems with edge IoT is ideal when real-time decision-making is required. Situating the AI system at the edge rather than in the cloud can effectively reduce latency to near zero.
For example, using machine learning (ML) algorithms to process IoT data at the edge in a driverless car could be a matter of life and death. In manufacturing, real-time data processing may distinguish between catastrophic equipment failure and timely preventive maintenance.
This also applies to optimisation, such as altering a machine’s speed or movement in real-time for precision manufacturing. An edge-based AI system can transmit instructions to equipment faster than a cloud-based system.
However, it’s not just about sheer speed and performance. Edge computing also offers enhanced security.
Cloud computing can present security risks associated with sending and retrieving data to the cloud. Conversely, edge computing can mitigate risks by filtering sensitive information at the source and storing it on-premise.
Moreover, facilities often have numerous mobile devices connected to the AIoT, handling substantial data. Transmitting all this data to the cloud may not be feasible, making edge analysis a better option. Edge analytics can extract high-value features from raw data, sending only crucial information to the cloud.
Use case: transportation
Driverless cars (and indeed other vehicles) have complex sensor stacks that ingest vast quantities of complex unstructured data, such as video and audio. Once this data enters the vehicle, it must be processed by edge AI decision-making devices.
For example, suppose a cow walks onto the road. The vehicle’s cameras feed that data to an edge AI processor, which uses ML algorithms to identify the obstruction and trigger the emergency brake. The whole process must be completed in mere milliseconds – there’s no time to send the video data to an external data centre for processing.
Adlink highlights their AVA-5500, an edge AI device designed to support railway hazard detection and automated train operation. IoT hardware and AI decision-making are deployed at the edge, so there’s no need to transmit data to the cloud. Edge camera-based analytics are ideal for remote applications, like trains and oil rigs.
Use case: security systems
Security systems also deploy edge AI to identify potential threats or unusual activities without sending data to a cloud for analysis. This speeds up the response time and reduces the amount of data that needs to be transmitted, saving bandwidth.
Use case: healthcare
In the healthcare sector, edge AI is combined with various health devices and health monitors to provide real-time health tracking and analysis.
These devices can process data locally without relying on external processing. Studies highlight edge AI’s potential for real-time precision medicine and confer security benefits.
Use case: manufacturing
Predictive and preventive maintenance depends on timely decision-making, ideally in real-time.
Big industry players like Nvidia offer edge AI solutions for manufacturing to make Industry 4.0 a reality, where automated production lines predict and initiate maintenance.
While saving valuable milliseconds from decision-making processes may seem inconsequential, processing IoT data at the edge is also securer and saves bandwidth.
Andrew Nelson, an architect at technology consultancy Insight, told The Enterprisers Project, “The [production] line itself can be instrumented to predict issues with bearings, belts, motors, etc…If you can predict or triage the issues quickly, you can minimize the downtime” and potentially save significant ongoing costs.”
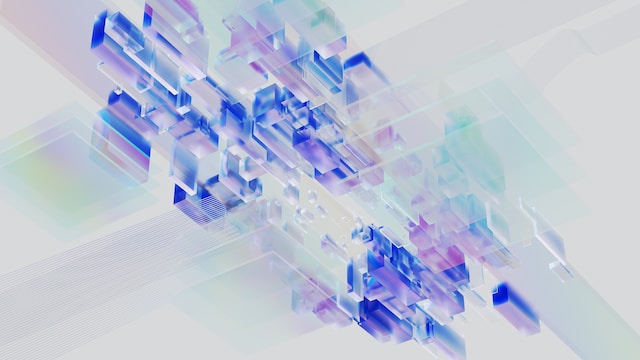
Benefits of using Edge AI in IoT
Integrating edge computing with IoT and AI offers many benefits that go beyond low-latency data processing:
- Increased speed and efficiency: By processing data locally, edge AI reduces the latency of sending data to the cloud, leading to faster decision-making and action.
- Reduced bandwidth: Edge AI reduces the need to send vast amounts of data over the internet, thereby saving bandwidth and reducing network congestion.
- Improved privacy and security: With edge AI, data can be processed locally on the device, reducing the risk of data being intercepted or tampered with during transmission.
- Operational resilience: Edge AI allows devices to operate independently of the cloud. This means that even if the network connection is lost, the device can continue to function, ensuring operational resilience.
Despite evident benefits, there are undoubtedly challenges to deploying this complex blend of IoT and AI technologies.
Challenges for adopting edge AI and IoT
Edge AI requires an ensemble of hardware and software working in concert. For instance, platforms like Crosser can IoT data in several ways before it reaches the AIoT processing. Data from several devices must be combined, cleaned and processed before passing into AI algorithms.
Moreover, edge AI platforms can also perform feature extraction to select relevant IoT data for algorithms to process.
Depending on the ML model, additional features may need to be engineered from raw IoT data.
For example, edge devices may need to convert machine vibration data from a format collected by an IoT device to a format readily utilised by an AI algorithm.
Summary
The convergence of edge AI and IoT creates a new frontier in AIoT technology, offering unprecedented opportunities for real-time data processing and decision-making.
As the technology evolves, edge AI in IoT will become simpler to deploy and more usable across different industries, offering massive potential for commercial and industrial applications and may also revolutionise wearables and portable technology.
The benefits go beyond sheer speed and precision – edge AI also reduces reliance on clouds, frees up bandwidth, and offers enhanced security.